A hacker has leaked 12,000 alleged Twilio name data, together with telephone numbers and audio recordings. The breach exposes private information, creating vital privateness dangers for companies and people utilizing the service.
A hacker, who has been fairly energetic just lately and goes by the alias ‘grep,’ has leaked over 12,000 (11,802) name data with audio, which they declare belong to Twilio clients. The leak’s timestamp ranges from 2019-06-14 to 2024-09-23.
Twilio is a San Francisco, California-based cloud communications platform that allows builders to combine voice, messaging, video, and authentication options instantly into purposes utilizing its API. As of 2024, the corporate has over 350,000 energetic buyer accounts, which implies the most recent alleged leak is roughly 3.37% of the overall accounts.
In keeping with the hacker, the alleged breach befell in September; nevertheless, they didn’t specify whether or not this was a third-party breach or if the data had been accessed instantly from Twilio’s methods.
The Hackread.com analysis staff managed to research the total leaked information. The leaked data are divided into two TXT information. The primary file seems to be a log of calls, doubtless extracted from a telecommunications system.
Each information monitor calls, doubtless within the context of a telecommunication and interpretation service. File 1 is a fundamental name log, whereas File 2 has extra particulars particular to interpretation providers, together with languages, charges, and session particulars. Right here’s a breakdown of the fields and the info it incorporates:
File 1
Start_time
& End_time
:
- These fields retailer the timestamps indicating when the decision began and ended.
- Instance:
'2019-06-14 05:40:49.924214'
to'2019-06-14 05:42:19.907792'
.
Called_from
& Called_to
:
- The telephone numbers of the caller (
called_from
) and the recipient (called_to
). - Instance:
+165********
is looking+144********
.
Standing
:
- Signifies the decision’s standing, reminiscent of whether or not it was ‘completed’, ‘failed’, or ‘no answer’.
- Instance:
'accomplished'
.
Period
:
- The period of the decision is in seconds.
- Instance:
90
seconds.
Notes
:
- It seems to be a placeholder for any extra feedback or notes associated to the decision.
- Generally, this discipline is empty.
Agent_id
, interpretation_session_id
, Call_sid
, course
, Call_type
, interpreter_id
: ID for the interpreter dealing with the decision and appears to be metadata.
Agent_id
: ID of the agent dealing with the decision.
Interpretation_session_id
: ID associated to an interpretation session (suggests this will likely contain translation providers).
Call_sid
: Distinctive identifier for the decision.
Route
: Whether or not the decision was ‘inbound’ or ‘outbound’.
Call_type
: Describes the kind of name, reminiscent of ‘incoming’ or ‘interpreter outbound’.
File 2
The second file appears to include extra detailed data for calls, probably involving interpretation or translation providers. It contains a wide range of extra fields for monitoring varied facets of the decision. Right here’s a breakdown:
Start_time
& end_time
:
- Identical as in File 1, displaying the beginning and finish time of the decision.
Called_from
& called_to
:
- Telephone numbers of the caller and recipient, similar to in File 1.
Standing
:
- Expanded statuses, reminiscent of ‘interpretation completed’ or ‘no answer’.
- Instance:
'interpretation accomplished'
.
Incoming_call_sid
:
- The identifier for the incoming name. This might assist map calls to their corresponding logs from File 1.
Period
:
- Period of the decision in seconds, much like File 1.
Language
:
- Specifies the language being interpreted through the name.
- Instance:
'English'
,'Arabic'
.
Price
:
- Attainable cost charge or interpreter value charge for the decision.
- Instance:
'1.00'
.
Interpretation_start_time
:
- Signifies when the precise interpretation session began.
- Instance:
'2019-06-14 05:42:02.498291'
.
Recording_url
:
- This discipline could retailer the URL for accessing a recording of the decision (though it’s usually
NULL
within the pattern information).
Agent_answered_time
, payout
, total_cost
:
- Metadata that tracks when the agent answered, and the monetary particulars (e.g., payout for the interpreter and whole value of the decision).
- Twilio hyperlinks with precise recording audio calls of consumers
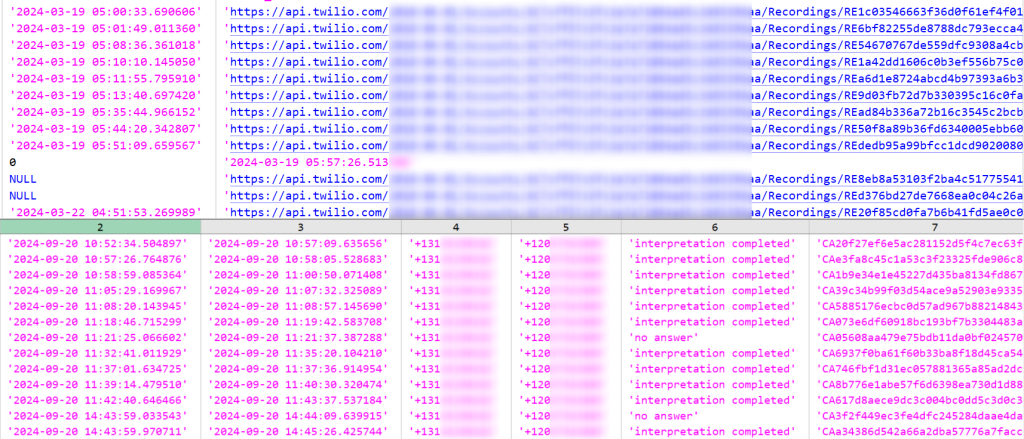
Conference_name
, sms_callback
, client_hold_time
, department_code
:
- Extra metadata fields associated to conferences, callbacks, and division monitoring.
Queued_count
:
- Tracks what number of instances the decision has been queued.
Is_video
:
- Signifies whether or not the decision was a video name.
- Instance:
0
(No video).
Remarks
, disposition_response_agent
:
- These fields could seize agent responses, name tendencies, or different follow-up actions.
Audio Name Recodings and Cybersecurity Dangers
Though the leaked information by quantity is minimal, the presence of precise name recordings significantly will increase the severity of the breach, because it exposes not simply metadata however the contents of conversations.
This may result in critical privateness violations, particularly if the calls contain delicate private or enterprise info. Attackers may exploit these recordings for blackmail, fraud, or impersonation. Companies may face authorized penalties beneath laws like GDPR or CCPA for failing to guard this information.
The leaked telephone numbers are additionally weak to smishing (SMS phishing) and vishing (voice name phishing) scams. Each strategies of assault are in style amongst cybercriminals and menace actors, significantly these supported by organized teams. A current instance is final month’s assault on companies in the US, the place hackers had been discovered calling staff to acquire their firm’s VPN credentials.
However, the scenario could require speedy actions together with notifying affected events, securing the leaked recordings, and consulting authorized specialists. Organizations must also overview entry controls, implement encryption, and contain an incident response staff to handle the breach.
RELATED TOPICS
- ShinyHunters Leak 33 Million Twilio Authy Telephone Numbers
- Telephone numbers of 42 million Iranians bought on a hacking discussion board
- Database of 176 million Pakistani cell phone customers bought on-line
- AT&T Knowledge Breach: Hackers Steal Name, Textual content Information for All Customers
- Names & Telephone numbers of 267 million Fb customers uncovered